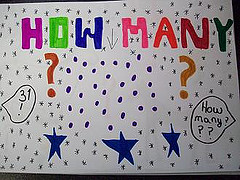
Prospective clients for our audience segmentation research often ask how many segments they’ll have, and if we know ahead of time what defines them.
We don’t. We don’t know what characteristics will define your audience segments, what’s going to be important in the segmentation and what isn’t, or even how many segments we’ll find.
That is different than some approaches, in which either the segment descriptors are predetermined (i.e. women vs. men, income levels, customers vs. non-customers, etc.) or others in which the analysis takes specific respondents as “seeds” — i.e. representative of the kind of people that you believe make up each of your segments — and then finds out how many other respondents are like each of those “seeds”. This approach doesn’t do anything to validate whether the descriptors defining the segments are important or valid — just tells you how many people fit that profile you’ve established.
Because our work is designed to explore the attitudes out there as opposed to validate existing assumptions about them, our analysis doesn’t identify a “seed” — it basically throws all the respondents and all their ratings on the attitudinal questions into a big pot, and does a bunch of calculations to determine which groups of attitudes clump together among significantly large groups of people to be important.
When we’re done, each of the respondents in the survey is assigned a “segment”. Each segment is defined by a collection of attitudinal elements, and everyone in each segment has ratings for all of those elements that are similarly interrelated. In short, there’s a strong mathematical matrix of connections between the segment members and the segment attitudinal elements.
The name for the type of analysis we do is “Orthogonally rotated factor analysis”, if you want to look it up. It requires a substantial level of statistical expertise to parse out the appropriate number of segments, which is done using a variety of additional statistical techniques within the process, which is why the person doing this work has a PhD in statistics.
In the end, we are seeing what the data says — what is important in defining these groups, how much of the audience is in each segment, and how many segments make sense given the statistical analysis.
We also avoid introducing any unnecessary bias by ensuring that the segmentation analysis is conducted without the analyst knowing what the actual attitudinal statements are, and without knowing any additional information about the respondents (e.g. demographics, product usage, etc.) Literally the ONLY thing that goes into the factor analysis is the numeric rating for each respondent on each of the attitudinal questions we asked — the respondents aren’t identified, the attitudinal questions are indicated only by number, and no other data collected in the survey is provided to the analyst doing the analysis. So that way the decisions about the segmentation are based purely on mathematical considerations.
There are many, many approaches to audience segmentation, and lots of debate about the right approach for particular applications. We believe in seeing what the numbers tells us, and taking it from there.
Leave a Reply